Review Article
Synthetic Animal: Trends in Animal Breeding and Genetics
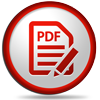
Abolfazl Bahrami1* and Ali Najafi2
1Department of Animal Science, University College of Agriculture and Natural Resources, University of Tehran, Karaj, Iran
2Molecular Biology Research Center, Baqiyatallah University of Medical Sciences, Tehran, Iran
*Address for Correspondence: A Bahrami, Department of Animal Science, Tehran University, Karaj, I.R. Iran, Tel/Fax: +98 9199300065; Email: a.bahrami@ut.ac.ir
Dates: Submitted: 31 December 2018; Approved: 10 January 2019; Published: 11 January 2019
How to cite this article: Bahrami A, Najafi A. Synthetic Animal: Trends in Animal Breeding and Genetics. Insights Biol Med. 2019; 3: 007-025. DOI: 10.29328/journal.ibm.1001015
Copyright License: © 2019 Bahrami A, et al. This is an open access article distributed under the Creative Commons Attribution License, which permits unrestricted use, distribution, and reproduction in any medium, provided the original work is properly cited.
Keywords: Synthetic biology; Systems biology; Synthetic approaches; Genetic engineering
Abstract
Synthetic biology is an interdisciplinary branch of biology and engineering. The subject combines various disciplines from within these domains, such as biotechnology, evolutionary biology, molecular biology, systems biology, biophysics, computer engineering, and genetic engineering. Synthetic biology aims to understand whole biological systems working as a unit, rather than investigating their individual components and design new genome. Significant advances have been made using systems biology and synthetic biology approaches, especially in the field of bacterial and eukaryotic cells. Similarly, progress is being made with ‘synthetic approaches’ in genetics and animal sciences, providing exciting opportunities to modulate, genome design and finally synthesis animal for favorite traits.
Introduction
Animal breeding
In 1859, Charles Darwin published his book ‘On the origin of species’, based on the findings that he collected during his voyage on ‘the Beagle’ [1]. He discovered the forces of natural selection. He also concluded that the individuals that fit best in their environment have the highest chance to survive and reproduce: they are the fittest. His conclusion was that the difference in food source, predators present, etc. between the islands had made develop differently over very many generations. Still, Darwin did not know about the basic laws of inheritance. It was the monk Gregor Mendel (https://history.nih.gov/exhibits/nirenberg/HS1_mendel.htm), who published the results of his studies of genetic inheritance in garden peas (https://history.nih.gov/exhibits/nirenberg/HS1_mendel.htm). He showed that genetic material is inherited from both parents, independently of each other. And that each (diploid) individual thus carries 2 copies of the same gene, of which only 1 is passed on to their offspring. Which one is a result of chance (independent assortment). He also showed that these gene copies (alleles) can be dominant (only 1 copy determines the expression of the gene), recessive (2 copies are required for expression), or additive (a copy of both alleles result in an expression that is intermediate to that of having 2 copies of either of the alleles). These findings had no immediate impact on animal breeding and were not recognized as important until 1900.
Most of the animal breeding and genetics theory we are still using today, was invented in the first half of the 20-th century. The statistician R. A. Fisher showed that the diversity of expression of a trait could depend on the involvement of a large number of so-called Mendelian factors (genes) [2]. Fisher, together with Sewall Wright and J.B.S. Haldane were the founders of theoretical population genetics [3,4]. Thomas Hunt Morgan and coworkers connected the chromosome theory of inheritance to the work by Mendel and created a theory where chromosomes of cells were believed to carry the actual hereditary material [5]. Jay L. Lush who is known as the modern father of animal breeding and genetics [6]. He advocated that instead of subjective appearance, animal breeding should be based on a combination of quantitative statistics and genetic information. The estimated breeding value (EBV) was only developed later by the statistician C. R. Henderson [7]. The estimated breeding value made it possible to rank the animals according to their estimated genetic potential (the EBV), which resulted in more accurate selection results and thus a faster genetic improvement across generations. Henderson further improved the accuracy of the estimated breeding value by deriving the best linear unbiased prediction (BLUP) of the EBV in 1950, but the term was only used since 1960. He also suggested to integrate the full pedigree of the population to include genetic relationships between individuals.
Candidate gene
The candidate gene approach to conduct genetic association studies focuses on associations between genetic variation within disease states or phenotypes and genes of interest. Candidate genes are often selected for study based on a priori knowledge of the gene’s biological functional that affected the traits or disease. Suitable candidate genes are generally selected based on known physiological, biological, or functional relevance to the traits in question. This approach is limited by its reliance on existing knowledge about known or theoretical biology of trait. However, more recently developed molecular tools are allowing insight into trait and disease mechanisms and pinpointing potential regions of interest in the genome. Many studies have used candidate genes as part of a multi-disciplinary approach to examining a phenotype or trait [8,9].
Genomic selection
Current great minds that have developed a way to incorporate large scale DNA information that has become available in animal model (BLUP) theory to estimate the so-called genomic breeding values are Theo Meuwissen and Mike Goddard [10]. Until 1953, scientists used statistics and presumed mechanisms to make predictions about inheritance. Nobody knew what exactly was the mechanism behind it. But in 1953, was discovered the double helix structure of DNA. In the beginning studying the DNA was very labor intensive and thus also very costly. Nowadays robots can perform large scale genotyping e.g. of more than 60,000 genetic markers on thousands of individuals within very limited amounts of time. A genetic marker can be considered a kind of ‘flag’ on the genome.
Main idea behind genomic selection is the association between the DNA make-up and performance of animals can add to the estimated breeding value, or even replace it. You can select animals already at very early age and you don’t have to wait until they become adult Because you don’t have to wait until the phenotype can be measured on the animals anymore as you have the associated DNA information. You can also use this for traits that are difficult to measure such as disease related traits. It would be highly desirable if you would only need to infect a finite number of animals and evaluate their response to the infection, link that to their DNA, and use that estimated link to predict the sensitivity of other animals to that disease based on their DNA, without having to infect them. Thus genomic selection refers to the use of genome-wide genetic markers to predict the breeding value of selection candidates [11]. This method relies on linkage disequilibrium between the markers and the polymorphisms that afford variation in important traits. Consequently, a linear prediction equation can predict the cumulative effect of many causal variants on the breeding value of the animal. Because it is possible to genotype individuals for 100000s of SNPs at a reasonable cost, the markers used in genomic selection are most commonly SNPs [12]. The equation that predicts breeding value from SNP genotypes must be estimated from a sample of animals, known as the reference population, that have been measured for the traits and genotyped for the SNPs. This prediction equation can be used to predict breeding values for selection candidates based on their genotypes alone. The candidates are ranked on these estimated breeding values, and the best ones are selected to breed the next generation [13].
The advantage and challenge of genomic selection
The advantage of genomic selection rather than traditional selection is that animals can be selected accurately early in life and for traits that are difficult or expensive to evaluate: disease resistance, fertility, feed conversion and methane emissions are prime examples. In dairy cattle, dairy bulls are traditionally selected pursuant progeny testing, because genetic merit for milk production of a bull can only be accurately measured through the milk production of his daughters. Progeny testing results in accurate selection, but with a generation interval of 5 years or longer. With genomic selection, the generation interval can be reduced to 2 years, potentially resulting in a 60–120% increase in the rate of genetic gain [14, 15]. However, genomic prediction across breeds has been largely unsuccessful to date, with prediction equations derived in one breed giving low accuracies in other breeds [16]. This is likely because of differences in linkage disequilibrium phases between SNPs and causative mutations. So maybe whole-genome sequence data may improve accuracy across breed prediction. The major challenge in applying genomic selection to some traits are important in the future, assembling large enough reference populations to make accurate predictions, because thousands to tens of thousands of phenotyped individuals are required [17,18].
Whole-genome sequencing
Although genomic estimated breeding values are now widely used as the foundation for choice of animals, there are some constraints of the current technology. It has become clear that much of the accuracy of genomic breeding values (based on 50000 DNA markers) in fact derives from prediction of the effect of large chromosome segments that segregate within closely related animals [19]. In this condition, the accuracy of the prediction equation will rapidly decay over generations as large chromosome segments break up because of recombination. Within breeds, effective population sizes are generally <200 and, consequently, animals within a breed have recent common ancestors and so apportion large chromosome segments. Using genomic predictions from whole-genome sequence data, may overcome some of these problems. Given that the causative mutations are present in the sequence data, the issue of decay in associations between causative mutations and SNP, which results in the decline in accuracy over time, may be overcome. Although this has been demonstrated in simulated data, in practice, to gain this will need a carefully designed reference population [10]. This requires a population in which the linkage disequilibrium between causative mutations and other variants is as limited as possible: if the extent of linkage disequilibrium is too large, the genomic prediction algorithms will distribute the effect of the causative mutation over variants across large chromosome segments, leading to the problem described above. If full genome sequence data could be used in genomic predictions rather than SNP arrays, because the causal mutations are in the data set, the accuracy is no longer bounded by linkage disequilibrium between SNP and causative mutations. Though the cost of genome resequencing has declined dramatically, it is too expensive to resequence the tens of thousands of individuals that would be required to estimate accurately the small effects of the large number of mutations affecting typical complex traits yet. In silico resequencing of large numbers of animals with specific phenotypes and the accumulation of these data across breeds would then enable highly accurate genomic predictions from whole-genome resequencing data. Breathtaking development is the sequencing of more dairy sires as part of the 1000 Bull Genomes Project, which is now underway [20]. Although using sequence data in genomic predictions is absorbing for the reasons described above, an important challenge will be the large number of SNPs and other variant effects to be estimated, with a still-limited number of records. The numbers of variants are likely to be in the tens of millions. One strategy to deal with this will be to use biological information such as “omics” data.
Results of animal breeding and genetics
Selective animal breeding already has about 300 years of history. A lot has been achieved since. Obvious results have been achieved in the field of cattle breeding. For example the Results obtained in cattle breeding: The increase until 1970 is much less steep than that from 1990 onwards. Reasons for this are many, but important ones are very strong increase in use of AI so that stronger selection in bulls was possible, introduction of more accurate techniques for estimating breeding values, introduction of automatic milking and the free stall instead of the tied stall, and better quality nutrition. The increase in phenotypic milk production in the period 1995 – 2013 is very similar to the estimated increase in genetic potential for milk production: approximately 1500 kg. This indicates that systematic improvements in the environment such as automatic milking, loose housing, and diet quality has similar effects on all cows.
Transgenic animal
Transgenic animals carry on embody one of the most exciting research tools in the biological sciences. Transgenic animals show unique models that are custom tailored to address specific biological questions. Hence, the ability to introduce functional genes into animals provides a very powerful tool for analyzing complex biological systems and processes. Gene transfer is of particular value in those animal species, where long life cycles reduce the value of classical breeding practices for rapid genetic modification. In general, a Transgenic Organism (TO) is any organism whose genetic material has been modified using genetic engineering techniques. This is an organism whose genetic makeup has been modified by the addition of genetic material from an unrelated organism. Transgenic involves the insertion, or deletion and mutation of genes. Inserted genes usually come from a different species in a form of horizontal gene-transfer. In nature this can happen when exogenous DNA interpenetrates the membrane of cell for any reason. This can be done artificially by physically inserting the extra DNA into the nucleus of the intended host with a very small syringe, attaching the genes to a virus, firing small particles from a gene gun and using electroporation [21,22]. Other methods exploit natural forms of gene transfer, such as the ability of lentiviruses to transfer genes to animal cells and the ability of Agrobacterium to transfer genetic material to plants [23,24]. Various development in genetics permitted humans to change the DNA and genes of organisms. Jackson et al. (1972) created the first recombinant DNA molecule when he combined DNA from a monkey virus with that of the lambda virus.25 The first transgenic livestock were produced and the first animal to synthesise transgenic proteins in their milk were mice, engineered to produce human tissue plasminogen activator.26-28 The first transgenic animal to be approved for food use was AquAdvantage salmon. The salmon were transformed with a growth hormone-regulating gene from a Pacific Chinook salmon and a promoter from an ocean pout enabling it to grow year-round instead of only during spring and summer [29]. TOs are used in production of drugs agriculture and experimental medicine with developing uses in conservation [30]. The first transgenic animal was created by injecting DNA into mouse embryos then implanting the embryos in female mice [31]. Transgenic animals currently being expanded can be placed into different broad classes based on the intended goal of the transgenic including to research human diseases, to produce products intended for human therapeutic use, to produce industrial or consumer products, to enhance production or food quality traits, to enrich or enhance the animals’ interactions with humans and to improve animal health. Dolly was a sheep and the first animal to be cloned from an adult somatic cell. Genetically modified animals are used as experimental models to test in biomedical research and for performing phenotypic [32]. Transgenic animals are becoming more vital to the discovery and development of treatments for many diseases. By changing the DNA or transferring DNA to an animal, we can create proteins that may be used in medical cure. Stable expressions of human proteins have been created in many animals, including pigs, sheep and rats. For example Human-alpha-1-antitrypsin, which has been tested in sheep and is used in treating humans with this deficiency and transgenic pigs with human-histo-compatibility have been studied in the hopes that the organs will be suitable for transplant with less chances of rejection [33]. Scientists announced that they had successfully transferred a gene into a primate species and made a line of breeding genetically modified primates for the first time [34]. Chinese scientists created dairy cows with genes from human beings to produce milk that would be the same as human breast milk [35]. Researchers from New Zealand also developed a transgenic cow that produced allergy-free milk [36].
Technologies to make transgenic animals
DNA microinjection: The favorable gene is injected in the pronucleus of a reproductive cell using a glass needle. The retouched cell is cultured in vitro to expand to a specific embryonic phase, is then transferred to a recipient female. DNA microinjection does not have a high efficiency, even if the new DNA is combined in the genome, the new traits will not appear in their offspring, if it is not accepted by the germ-line [37].
Retrovirus-mediated gene transfer: A retrovirus is a virus that moves its genetic material in the form of RNA instead of DNA. Retroviruses are used as vectors to transfer genetic material into the host cell. The result is a chimera, an organism include parts or tissues of diverse genetic constitution [37].
Restriction enzyme mediated integration: Restriction enzyme mediated integration (REMI) is a technique for combining DNA into the genome sites that have been created by the same restriction enzyme used for the DNA linearisation. The plasmid combine occurs at the corresponding sites in the genome, often by regenerating the diagnosis sites by same the restriction enzyme used for plasmid linearization [37].
Stem cell transgenesis
Multipotent: Multipotent stem cells can only differentiate into a finite number of therapeutically beneficial cell types, however their safety and relative lack of complication to us have resulted in the extensive majority of personalized cellular therapeutics involving multipotent stem cells [38].
Pluripotent: Transgenic vectors can be hand over randomly or targeted to a particular genomic location, such as a safe harbor. Scientists have done research and technology improvement to provide the tools necessary to allow effective and safe pluripotent stem cell (PSC) transgenesis [39,41].
Totipotent: The administered gene is inserted into totipotent stem cells, cells which can expand into any specialized cell. Cells containing the desirable DNA are combined into the host’s embryo, resulting in a chimeric animal. Unlike the other two methods which need to live transgenic offspring for testing, embryonic cell transfer can be examined at the cell stage [42,43].
Genome editing
Genome editing with engineered nucleases (GEEN) is a kind of genetic engineering in which DNA is replaced, inserted or deleted in the genome of an organism by using engineered nucleases. These nucleases create site-specific double-strand breaks (DSBs) at desirable locations in the genome. The induced double-strand breaks are repaired through homologous recombination (HR) or nonhomologous end-joining (NHEJ), resulting in targeted mutations. Currently, there are four families of engineered nucleases that being used: zinc finger nucleases (ZFNs), meganucleases, the CRISPR-Cas system and transcription activator-like effector-based nucleases (TALEN) [44]. Among the most important requirements of reverse genetic analysis is the ability to manipulate the DNA sequence of the target organism. This can be arrived by:
• Recombination based methods that use the natural ability of cells to swap DNA between an exogenous DNA and its own genetic information.
• Site-directed mutagenesis hiring either polymerase chain reaction (PCR) or phage- mediated methods and oligonucleotides containing the desired mutation [45].
• Drawbacks of these approaches
• Phage and PCR -mediated approaches are less successful in more complicated organisms such as mammals, where delivery becomes more difficult.
• Recombination-based methods can be inefficient.
• They also need to stringent choice steps and thus the addition of selection-specific sequences, along with those incorporated into the DNA [46].
Double stranded breaks
Basic to the use of nucleases in genome editing is the meaning of DNA double stranded break repair mechanisms. The known DNA double stranded break repair pathways that are functional in all organisms are homology directed repair (HDR) and the non-homologous end joining (NHEJ).
Site-specific double stranded breaks
Development of a DNA double stranded break in DNA should not be a challenging task as the used restriction enzymes are capable of doing so. However, if genomic DNA is treated with a specific restriction endonuclease many DNA double stranded breaks will be made. This is a result of the fact that most restriction enzymes identify a few base pairs on the DNA as their target and very likely that specific base pair composition will be found in many locations across the genome. To overcome this challenge and make site-specific DNA double stranded break, three different classes of nucleases have been discovered. These are the transcription-activator like effector nucleases (TALEN), Zinc finger nucleases (ZFNs) and meganucleases. Below is a brief overview of these enzymes.
Meganucleases have the unique feature of having long recognition sequences thus creating them naturally is very specific [47]. This can be exploited to make site-specific DNA double stranded break in genome editing; however, the challenge is that known meganucleases are insufficient to cover all possible target sequences. To dominate this challenge, mutagenesis and high throughput screening methods have been used to make meganuclease variants that identify unique sequences Others have been able to fuse various meganucleases and make hybrid enzymes that identify a new sequence [48,49].
Meganucleases have the profit of causing less toxicity in cells than methods such as zinc finger nucleases, likely because of more stringent DNA sequence recognition; Although, the manufacturing of sequence-specific enzymes for all possible sequences is time consuming and costly, as one is not profiting from combinatorial possibilities that methods such as zinc finger nucleases and the transcription-activator like effector nucleases-based fusions utilize [47]. In spite of meganucleases, the concept behind zinc finger and the transcription-activator like effector nucleases technology is based on a non-specific DNA cutting enzyme, which can then be linked to special DNA sequence recognizing peptides such as transcription activator-like effectors (TALEs) and zinc fingers. The most important for this was to find an endonuclease whose DNA recognition site and cleaving site were separate from each other, a location that is not common among restriction enzymes [50]. A restriction enzyme with such properties is FokI. Additionally FokI has the advantage of need dimerization to have nuclease activity and this means the specificity increases dramatically as each nuclease partner would identify a unique DNA sequence. To increase this effect, FokI nucleases have been modified that can only function as heterodimers and have increased catalytic activity [51]. Though the nuclease portions of both zinc finger and the transcription-activator like effector nucleases constructs have the same properties, the difference between these engineered nucleases is in their DNA recognition peptide. Zinc finger nucleases rely on Cys2-His2 zinc fingers and the transcription-activator like effector nucleases constructs on TALEs. Both of these DNA recognizing peptide domains have the characteristic that they are found in compositions in their proteins. Cys2-His2 Zinc fingers typically happen in repeats that are 3 bp apart and are found in diverse combinations in a variety of nucleic acid interacting proteins such as transcription factors [47]. One recent improvement integrates the DNA binding specificity of transcription activator-like effectors with the nuclease specificity of meganucleases; these “megaTALs” are fit with all current technologies and may represent improvements on existing methods [52].
Systems biology
Systems biology is the mathematical and computational modeling of complicated biological systems. An appearing engineering approach applied to biological research, systems biology is a biology-based interdisciplinary field of survey that focuses on complicated interactions within biological systems, using a comprehensive approach to biological research. Exclusively from year 2000 onwards, the concept has been used widely in the biosciences in a variety of grounds. One of the developmental aims of systems biology is to model and discover emergent attributes, properties of organisms, tissues and cells functioning as a system whose theoretical description is only possible using techniques which fall under the shrink of systems biology. These typically involve cell signaling or metabolic networks [53,54].
Different aspects of system biology:
• As a field of study of the interactions between the components of biological systems, and how these interactions increase to the behavior and function of that system [55].
• As a series of usable protocols used for doing research, namely a cycle composed of theory, experimental validation, analytic or computational modelling to offer specific testable hypotheses about a biological system and then using the recently acquired quantitative description of cell processes or cells to refine the computational model [56]. Since the purpose is a model of the interactions in a system, the experimental techniques that most suit systems biology are those that are system-wide and effort to be as complete as possible. Thus, transcriptomics, proteomics, metabolomics and high-throughput techniques are used to gather quantitative data for the construction and validation of models [57].
• As the usage of dynamical systems theory to molecular biology. Indeed, the concentrate on the dynamics of the studied systems is the principal conceptual difference between bioinformatics and systems biology. Ludwig von Bertalanffy who can be seen as one of the pioneers of systems biology with his systems theory [58]. One of the first numerical simulations in cell biology was published by neurophysiologists Alan Lloyd Hodgkin and Andrew Fielding Huxley, who constructed a mathematical model that explained the function potential propagating along the axon of a neuronal cell [59]. Denis Noble (1960) expanded the first computer model of the heart pacemaker [60].
The formal study of systems biology, as a distinguished discipline, was started by systems theorist Mihajlo Mesarovic entitled “Systems Theory and Biology” [61]. The successes of molecular biology throughout the 1980s, coupled with doubt toward theoretical biology, that then promised more than it achieved, effected the quantitative modelling of biological processes to become a slightly minor field [62]. However the birth of functional genomics in the 1990s meant that large quantities of high quality data became available making more realistic models possible. Several articles on systems genetics, systems medicine and systems biological engineering were published [63-65]. The group of Masaru Tomita published the first quantitative model of the metabolism of a whole cell [66]. Systems biology emerged as a movement in its own right after Institutes of Systems Biology were established in Seattle and Tokyo, spurred on by the completion of various genome projects, the large increase in data from the omics and the accompanying advances in bioinformatics and high-throughput experiments. In 2002 and 2003, the some foundations and institutions put forward a grand challenge for systems biology to construct a mathematical model of the whole cell. In 2006, because of a shortage of people in systems biology several doctoral programs in systems biology have been established in many parts of the world. The first whole-cell model of Mycoplasma Genitalium was achieved in 2012. The whole-cell model is able to predict viability of Mycoplasma Genitalium cells in response to mutations [67]. Pursuant to the explanation of systems biology as the ability to gain, integrate and analyze complicated data sets from multiple experimental sources using interdisciplinary tools and databases (Tables 1,2), some typical technology platforms are: Genomics, Transcriptomics, Epigenomics or Epigenetics, Translatomics or Proteomics, Metabolomics, Phenomics, Interferomics, Glycomics, Lipidomics, Interactomics, NeuroElectroDynamics, Fluxomics, Biomics, Semiomics and Cancer Systems Biology. The systems biology approach often involves the expansion of mechanistic models, such as the reconstruction of dynamic systems from the quantitative properties of their elementary building blocks. Because of the large number of parameters, constraints and variables in cellular networks, computational and numerical techniques are often used for example flux balance analysis (FBA) [68].
Synthetic biology
Synthetic biology is an interdisciplinary field of engineering and biology. The subject incorporates various disciplines from within these domains, such as evolutionary biology, biotechnology, molecular biology, biophysics, computer engineering, genetic engineering and systems biology. Definition, by Jan Staman, described it as “a new emerging scientific field where ICT, biotechnology and nanotechnology meet and strengthen each other” (http://www.synbiosafe.eu/uploads). Synthetic biology description is designing and constructing biological modules biological systems, and biological machines for useful purposes [69]. Progress is being made with synthetic approaches in genetics and animal sciences, providing exciting opportunities to modulate, genome design and finally synthesis animal with favorite traits. Thus in this paper we have explained and illustrated applications of synthetic biology specially to animal breeding and genetics. A notable advance in synthetic biology occurred when two articles by Michael B. Elowitz and Stanislas Leibler discussed (in 2000) the creation of biological circuit devices of a genetic toggle switch and a biological clock by combining genes within Escherichia coli cells [70,71]. Studies in synthetic biology can be subdivided into broad assortments according to the approach they take to the problem at hand: biomolecular engineering, standardization of biological parts, genome engineering and genome design [72]. Because of the complication of natural biological systems, it would be simpler to rebuild the systems of interest from the ground up; until provide engineered surrogates that are easier to understand, control and manipulate [73]. Tables 1 and 2 show the collection of a list of tools, databases and methods for synthetic biology.
Table 1: This table contains a collection of a list of tools, databases and methods for synthetic biology. | |
Tools, databases and methods for synthetic biology | Discretion |
BBOCUS | A re-implementation of the algorithm in Graziano Pesole's BACKTR. It's based on cluster analysis (Complete Linkage algorithm), that requires a similarity matrix D containing distance between each pair of sequences of mRNA |
Benchling | Free online tools for vector editing, restriction analysis, primer search, multi-sequence alignment, and more |
Biopolymer calculator | Calculate extinction coefficients, Tm's, and base composition for your DNA or RNA; calculate amino acid composition and extinction coefficient for your protein |
Clipboard | Web tool for getting complement, reverse complement, translation and restriction enzyme analysis of a DNA sequence |
Cytostudio | An integrated development environment and a compiler for a high-level bio-programming language for Synthetic Biology |
DNA Works | A web tool for optimizing melting temperature during gene synthesis. |
File format converter | Web tool for converting between sequence file formats |
Geneious | Comprehensive suite of tools for molecular biology |
Genome Compiler | The industry's most user friendly genetic engineering design tool |
GeneDesign | Collection of online (and some command line) tools for codon optimization and shuffling, restriction site editing |
GeneDesigner | Combine genetic building blocks by drag-and-drop, codon optimize, restriction site editing, sequence oligo design |
GenoCAD | A design tool that uses collections or libraries of genetic parts and explicit design rules describing how these parts should be combined to engineer genetic constructs. |
NEB Cutter | Tool for finding restriction sites |
Synthetic Gene Designer | A web platform that allows codon optimization to various extent |
Appendix | Website with many useful nucleic acid parameters |
Modeller | For homology or comparative modeling of protein three-dimensional structures |
Zinc Finger Tools | Design Zinc Finger DNA binding proteins |
TinkerCell | Construct computational models using biological parts, cells, and modules |
Metabolic Tinker | Construct thermodynamically feasible metabolic paths among user-defined compounds |
Registry of Standard Biological Parts | Open repository of BioBricks; the place for all your standard biological parts |
Gibson Assembly | Complementary termini of ~40 bp sequence homology determine the order in which substrate sequences are assembled |
Golden Gate, Golden Braid, and MoClo | Offers standardized, quasi-scarless, multi-part DNA assembly, and is an excellent choice for combinatorial library construction |
Infobiotics.org | A computational framework implementing a synergy between executable biology, multi-compartmental stochastic simulations, formal model analysis and structural/parameter model optimization for computational systems and synthetic biology |
Liverpool GeneMill | Offer open access to synthetic biology services through the new allied research facility |
Open Plant | A BBSRC/EPSRC Synthetic Biology Research Centre, supported by the Research Councils' Synthetic Biology for Growth programme. |
Pcomp | A database of rationally designed Peptide components for synthetic biology |
Synthetic Biology Open Language | Can be used to represent genetic designs through a standardized vocabulary of schematic glyphs (SBOL Visual) as well as a standardized digital format (SBOL Data) |
Table 2: This table contains a collection of a list of tools, databases and methods for system biology. | |
Tools, databases and methods for system biology | Discretion |
SBML | SBML is a software-independent language for describing models common to research in many areas of computational biology, including cell signaling pathways, metabolic pathways, gene regulation, and others |
Cell Designer | A modeling tool of biochemical networks |
Copasi | COPASI is a software application for simulation and analysis of biochemical networks and their dynamics |
Cytoscape | open source software platform for visualizing molecular interaction networks and biological pathways and integrating these networks with annotations, gene expression profiles and other state data |
SBML toolbox for MATLAB | SBML Toolbox provides functions for creating and validating models; and manipulating and simulating these models using ordinary differential equation solvers |
Stoch SS | an integrated development environment (IDE) for simulation of biochemical networks |
GNU MCSim | a simulation package, written in C |
BIOCHAM | The Biochemical Abstract Machine (Biocham) is a modelling environment for systems biology |
BioCharon | BioCharon, that integrates modeling and analysis tools for biomolecular networks |
BioSPICE Dashboard | an open source framework and software toolset for Systems Biology, is intended to assist biological researchers in the modeling and simulation of spatio-temporal processes in living cells |
Cellerator | extending a computer algebra system to include biochemical arrows for signal transduction simulations |
Cellware | A New Modeling and Simulation Tool for Modeling Cellular Transactions. |
INSILICO discovery | an advanced computational tool for network oriented "in silico" analysis and design of cellular properties |
Jarnac | A fast simulator of reaction networks |
JigCell | a modeling and simulation software that also enable parameter estimation |
JSIM | a Java-based simulation system for building and analyzing quantitative numeric models |
Kinsolver | a simulator for biochemical and gene regulatory networks |
MesoRD | Mesoscopic Reaction Diffusion Simulator |
MMT2 | Modeling and simulation software for metabolic networks |
PathwayLab | an in silico pathway analysis tool, enabling pharmaceutical R&D to reach their target decisions faster and with higher accuracy |
PNK 2e | a software environment for the modeling and simulation of biological processes |
PROTON | an Integrative Modeling System |
PySCeS | the Python Simulator of Cellular Systems |
runSBML | A pathway simulation tool by Ariadne Genomics |
SBML ODE Solver | a high pecision ODE solver for SBML |
SBMLSim | provides a Matlab GUI that allows the user to import a SBML model, simulate it, and visualize the simulations |
SigTran | a modeling environment especially designed to enable biological researchers to carry out large scale simulations and analysis of complex signal transduction networks |
Virtual Cell | Cellular simulation software |
BioUML | Java framework for systems biology |
The essential gene
Essential genes are those genes of an organism that are thought to be critical for its survival. Although, being essential is dependent on the conditions in which an organism lives. For example, a gene required to digest starch is only essential if starch is the only source of energy. More recently, systematic attempts have been made to detect and identify those genes that are completely required to maintain life, provided that all nutrients are available [74]. These experiments have led to the conclusion that the completely required number of genes for bacteria is on the order of about 250-300. These essential genes encode proteins to maintain a central replicate DNA, metabolism, translate genes to proteins, maintain a basic structure, and mediate transport processes into and out of the cell. Most genes are not essential but convey selective useful and increased fitness.
Determining the sets of genes necessary for survival of diverse organisms has helped to detect the fundamental processes that sustain life across an array of environments [75]. This study has also applied as the starting point for efforts by synthetic biologists to design organisms [76]. In spite of the importance of essential gene sets, they have traditionally been challenging to gather because of the difficulty of observing mutations that result in phenotypes. Recently, the pairing of transposon mutagenesis with next generation sequencing (NGS), referred to collectively as transposon sequencing (Tn-seq), has resulted in a dramatic advance in the detection of essential gene sets [77,78]. The important characteristic of Tn-seq is the use of high-throughput sequencing to screen for the fitness of every transposon mutant in a pooled population to measure each mutation’s effect on survival. This information can be used to quantitatively ascertain the impact of loss-of-function mutations at any given locus, intergenic or intragenic, in the conditions under which the library is grown [79]. Essential gene sets for 42 diverse organisms distributed across all three domains have now been defined [80]. A recently developed variation on Tn-seq, random barcode transposon site sequencing (RB-TnSeq), further minimizes the library preparation and sequencing costs of whole-genome mutant screens [81].
In spite of the proliferation of genome-wide essentiality screens, a complete essential gene set has yet to be defined for a synthetic organism. In algae, efforts are underway to produce a Tn-seq like system in Chlamydomonas reinhardtii; however, the mutant library currently lacks sufficient saturation to determine gene essentiality [82]. The absence of experimentally determined essential gene sets in organisms, despite their importance to the environment and industrial production, is largely because of the difficulty and time required for genetic modification of these organisms. As a result, it has been developed as a model organism and a production platform for a number of fuel products and high value chemicals [83].
DNA synthesis
It was reported that several group were offering the synthesis of genetic sequences up to 2000 bp long and a period time of less than 2 weeks. Nucleotides harvested from an inkjet manufactured DNA chip incorporate with DNA mismatch error-correction permits cheap large-scale changes of codons in genetic systems to improve gene expression or combined novel amino-acids.84 In Addition, the CRISPR/Cas system has appeared as a promising technique for gene editing. It was hailed as “the most important innovation in the synthetic biology space in nearly 30 years.” While other methods take years to edit sequences, CRISPR speeds that time up to weeks [84].
DNA sequencing
Synthetic biologists develop use of DNA sequencing in their work in several ways. Firstly, large-scale genome sequencing attempts continue to provide a worth of information on naturally occurring organisms. Theses information provides a wealthy substrate from which synthetic biologists can create devices and parts. Secondly, synthetic biologists apply sequencing to consider that they fabricated their engineered system as intended. Thirdly, speedy, inexpensive and reliable sequencing can also facilitate fast detection and identification of synthetic systems and organisms [85].
Modeling
Models apprise the design of biological systems by permitting synthetic biologists to better predict system manner prior to fabrication. Synthetic biology will profit from better models of how DNA encodes the information needed to determine the cell, how biological molecules bind substrates and catalyze reactions and how multi-component integrated systems act. Newly, multiscale models of gene regulatory networks have been developed that focus on synthetic biology usages. Simulations have been used that model all bimolecular interactions in transcription, translation, regulation, and induction of gene regulatory networks, guiding the design of synthetic systems [86-88].
Synthetic DNA
Dramatic decreases in expenditure of making nucleotides, the sizes of DNA making from oligos have increased to the genomic level [89]. For instance, researchers reported synthesis of the 9.6 kilo base pair of Hepatitis C virus genome from chemically synthesized 60 to 80-mers [90]. The 5386 base pair genome of the bacteriophage Phi was assembled in about 2 weeks [91]. The same group had made a synthetic genome of a novel minimal bacterium, M. laboratorium and were working on getting it functioning in a living cell [92].
Synthetic gene networks
Synthetic biological ON–OFF switches a set of genes can be choose and merged, to interact in a controllable and predictable manner, forming a system with a pre-set function also known as synthetic gene circuit. Than construct higher-order gene networks for advanced therapeutic applications, a toolbox of well-controllable standardized and well-characterized section should be available. In some cases, these simple gene networks are also used as therapies. For performing logical operations in cells, programmable Boolean logic gates were created in 2004, by incorporating heterogeneous transcription factors [93]. A gene network performed by a Boolean AND gate was applied for targeting cells where the AND gate activity was got when both pre-set situations were met, leading to the expression of apoptotic genes and cell death [94]. Boolean logic have also been engineered based on synthetic transcription factor (TF)-containing zinc finger motifs (ZF) and clustered regularly interspaced short palindromic repeats (CRISPR)/Cas9 motifs [95]. These are attractive sections for engineering higher-order networks because (i) CRISPR/Cas9 and zinc fingers can be made to recognize virtually any DNA sequence, (ii) they can function without interfering with each other. Indeed, the bacterial CRISPR/Cas system has been shown to be easy and versatile to use. Recently, Qi et al. (2013) showed that an endonuclease-deficient Cas9 can be used as a programmable ‘CRISPRi’ tool for gene silencing in E. coli [96]. Inhibitory circuits in mammalian cells have been introduced using dCAS9 systems [41,97]. Recently, CRISPR regulatory devices were layered to get cascaded circuits and the expression of functional guide RNAs (gRNAs) from RNA polymerase II promoters and multiplexed production of gRNAs and proteins from a single transcript in human cells was made possible [98,99]. In the discussed switches, the switching molecule should be present in order to maintain the switch in either the OFF or ON state. To reversibly set the switch to OFF or ON positions by applying a trigger molecule, toggle switches have been developed [100]. Examples of how these toggle switches have been occupied include the presence of hormones or signalling molecules or monitoring the environment of immune cells in lymph nodes. Though more complicated in network topology, functionally, synthetic mammalian oscillators constitute synthetic biological parts that can be unified into higher-order circuits or to govern metabolic, signalling pathways and repair in mammalian cells. Such a synthetic oscillator has been developed by using a time-delayed negative feedback loop, but these systems have been shown to dampen their oscillations because of noise and/or epigenetic silencing [101-105]. The addition of a positive feedback loop may dominate these limitations and generate autonomous and tune able oscillatory expression of reporter genes [105]. A low-frequency mammalian oscillator has also been developed, by silencing of the tetracycline-controlled transactivator using siRNA encoded in the introns of the mRNA, in order to facilitate robust and autonomous expression of a fluorescent reporter protein with periods of 26 h [106]. In order to generate transcriptional and translational time-delay for tuning oscillators, inteins could also be employed [107]. All of these synthetic biological control circuits described in this section contribute to the development of mammalian cell biocomputers [108].
Synthetic genomics
Synthetic genomics is an aborning field of synthetic biology that uses aspects of genetic modification on pre-existing life forms with the purpose of producing some product or desired manner on the part of the life form so created.
Researchers were able to build a synthetic organism for the first time. It was accomplished by synthesizing a 600 kilo base pair genome (resembling that of M. genitalium) via Transformation Associated Recombination and the Gibson Assembly method [109].
Synthetic life
One important subject in synthetic biology is synthetic life, artificial life made in vitro from biomolecules and their component materials. Synthetic life experiments try to either study some of the properties of life, probe the origins of life or more ambitiously to rebuild life from non-living components. Synthetic biology tries to create new biological molecules and even novel living species. In the area of synthetic biology, a living “artificial cell” has been defined as a completely synthetically-made cell that can maintain ion gradients, capture energy, contain macromolecules and have the ability to mutate [110]. The first living organism with ‘artificial’ DNA was produced as Escherichia coli was engineered to replicate an expanded genetic alphabet [111]. A completely synthetic genome was produced and introduced to genomically emptied bacterial host cells [112].
The ethics and public acceptance issues
A variety of potential harms are being recognized with synthetic biology and relate subject. One way to carve up these potential harms is to individualize between what we call “physical harms” and “non-physical harms.” These potential harms are not unique to synthetic biology or synthetic life, they are the same concerns that have been appointed in the context of other emerging technologies such as neuroscience, genetics, nanotechnology and stem cell research. In the literature, we observed justly consistent agreement about what might be the potential physical harms of synthetic biology, though there is disagreement about how likely those harms are to burgeon and about what action, if any and at what cost, should be taken for preventing them. Enthusiasts tend to adopt a pro-actionary approach to the hazard of physical harm, arguing that we should not search to interfere with the development of an appearing technology unless we have very good reason to suspect that it will cause serious physical harm. Alongside self-regulation, some enthusiasts defend the use of public funds for the kind of public engagement that seeks primarily to educate the public about risks and benefits so that members of the public can become informed consumers of emerging technologies. Critics (those who are concerned about advances) tend to adopt a pre-cautionary view, arguing that we should be prepared to interfere with the development of an emerging technology if we have good reason to suspect that it might cause serious physical harm, and they generally see such a risk in synthetic biology.
Critics defend for oversight, regulation and the kind of public engagement that shapes the development of emerging technologies, such as is practiced in some countries around genetically modified foods and other emerging technologies and is being employed and studied around nanotechnology [113-115]. Many people fall somewhere on the spectrum between critics and enthusiasts, finding themselves fuzzy between the insights of each side. On the question of non-physical harms, we observed some agreement among enthusiasts and critics that some nonphysical harms are discussing. While there is surely more work to do in identifying, conceptualizing and addressing these non-physical harms, there is already some acceptance, for example, of the legitimacy of the concern that patents might slow down research and of voluntary open-source practices as one way to address this concern. However, there are non-physical harms that have thus far received short shrift in discussion of synthetic biology. This group of non-physical harms centres around concerns about the suitable relationship between nature and humans and about whether humans must to create new kinds of life. We suggest that those who lead and fund synthetic biology search critically evaluate, and carefully describe, concerns about both physical and nonphysical harms. In so doing, they should draw on our experience of these concerns in the context of other emerging technologies, including neuroscience, genetics and nanotechnology. It will also be important, when examining concerns about physical and non-physical harms, to seek to carefully describe, and critically evaluate, the various understandings of these concerns and suggested responses to them that are formulated from within both the pre-cautionary and pro-actionary frameworks. We need to better understand what individuals in our society mean when they cite a concern that some synthetic biology or synthetic life is against nature (or is playing God). For those who believe that the job of human beings is to shape themselves and the rest of the natural world, synthetic biology is a clear next step, and concerns about “playing God” are incoherent. While powerful, that understanding of our place in the world is but one very specific understanding. For those who believe that the job of human beings is to accept and “let be” some features of themselves and the rest of the natural world those questions are worth taking seriously. By better understanding exactly what values are considered at play in the context of synthetic biology, we will be in a better circumstances to understand what action would be reasonable to recommend or expect. As with other harms, we should draw on our experience of these concerns in the context of other emerging technologies, including neuroscience, genetics and nanotechnology. Understanding and respect can affect the choice of experiments and eventual products, the communication of results and the direction of publicly funded programs. It can also make more receptive those who might initially have opposed synthetic biology.
Conclusion
Abstract of trends in animal breeding and genetics also some relate subject has been shown in figure 1. New and conventional genetic architecture can be defined using system biology information open opportunities for novel applications in animal breeding and genetics. Biomarkers of physiological states can be used to breed the best animals. Now, omics has been applied only to deal with genetic questions in a few species. Practical issues in collecting samples and implementing suitable experimental designs should be also considered, according to the sensitivity of omics profiles to environmental conditions. However, advancements in this field and synthetic biology are expected, moving the bottleneck on the interpretation and use of omics information in animal breeding and genetics for which new methodological developments will contribute to better define approach in the omics era. In addition, synthetic biology is an emerging interdisciplinary research field combining biology, computational science and mathematics, which aims at creating models for dynamic interactions of system components. Animal sciences have arrived at the threshold of a genomics data explosion. It is now in a position to make most effective use of the improved knowledge on the structure, variation expression and synthesis of animal genomes. The application of synthetic biology approaches using these omics information will provide better insight into the biology. Consequently, it will provide opportunities to monitor, modulate, and improve animal. Synthetic biology approaches require a close collaboration between many different disciplinary scientific communities that share resources, knowledge and technologies, and that are willing to integrate their data sets. With the development of synthetic biology approaches, we are entering the era of a predictive theoretical biology for farm animal as well as genetic manipulations.
Acknowledgement
We would like to acknowledge F. Marandi for his helpful feedback on the manuscript.
References
- Charles D. On the Origin of Species by Means of Natural Selection, or the Preservation of Favoured Races in the Struggle for Life. Nature. 1859; 5: 502. Ref.: https://goo.gl/Vd9Zda
- Wright S. Statistical genetics and evolution. Bull Amer Math Soc 1942; 48: 223–246. Ref.: https://goo.gl/kFP84Y
- Fisher RA. The Correlation between Relatives on the Supposition of Mendelian Inheritance. Philosophical Transactions of the Royal Society of Edinburgh. 1918; 52: 399–433. Ref.: https://goo.gl/1FTvrH
- Haldane JB. Linkage in poultry. Science. 1921; 54: 663. Ref.: https://goo.gl/ayCNS9
- Morgan TH. Sex-limited inheritance in Drosophila. Science. 1910; 32:120–122. Ref.: https://goo.gl/cpCDXv
- Lush JL. 1896 - 1982 Biographical Memoirs of the AAAS. Ref.: https://goo.gl/C133gz
- Van Vleck LD. Charles Roy Henderson, 1911-1989: A brief biography. J Anim Sci. 1998; 76: 2959–2961. Ref.: https://goo.gl/Gjhraa
- Bahrami A, Miraei-Ashtiani SR, Mehrabani-Yeganeh H. Associations of growth hormone secretagogue receptor (GHSR) genes polymorphisms and protein structure changes with carcass traits in sheep. Gene. 2012; 505: 379–383. Ref.: https://goo.gl/GZy8PK
- Bahrami A, Behzadi SH, Miraei-Ashtiani SR, Roh SG, Katoh K. Genetic polymorphisms and protein structures in growth hormone, growth hormone receptor, ghrelin, insulin-like growth factor 1 and leptin in Mehraban sheep. Gene. 2013; 527: 397–404. Ref.: https://goo.gl/sZu7RM
- Meuwissen TH, Goddard ME. Accurate prediction of genetic values for complex traits by whole-genome resequencing. Genetics. 2010; 185: 623–631. Ref.: https://goo.gl/2jynnU
- Meuwissen TH, Hayes BJ, Goddard ME. Prediction of total genetic value using genome-wide dense marker maps. Genetics. 2001; 157: 1819–1829. Ref.: https://goo.gl/CdLnVe
- Cole JB, VanRaden PM, O'Connell JR, Van Tassell CP, et al. Distribution and location of genetic effects for dairy traits. J Dairy Sci. 2009; 92: 2931–2946. Ref.: https://goo.gl/Jucx4Q
- Daetwyler HD, Kemper KE, van der Werf JH, Hayes BJ. Components of the accuracy of genomic prediction in a multi-breed sheep population. J Anim Sci 2012; 90: 3375–3384. Ref.: https://goo.gl/mhxhnB
- Pryce JE, Daetwyler HD. Designing dairy cattle breeding schemes under genomic selection: a review of international research. Anim Prod Sci. 2011; 52: 107–114. Ref.: https://goo.gl/tfrgDu
- Schaeffer LR. Strategy for applying genome-wide selection in dairy cattle. J Anim Breed Genet. 2006; 123: 218–223. Ref.: https://goo.gl/iCbuqF
- Erbe M, Hayes BJ, Matukumalli LK, Goswami S, Bowman PJ, et al., Improving accuracy of genomic predictions within and between dairy cattle breeds with imputed high-density single nucleotide polymorphism panels. J Dairy Sci. 2012; 95: 4114–4129. Ref.: https://goo.gl/XfWQiV
- Daetwyler HD, Villanueva B, Woolliams JA. Accuracy of predicting the genetic risk of disease using a genome-wide approach. PLoS ONE. 2008; 3: e3395. Ref.: https://goo.gl/JPHkUa
- Goddard M. Genomic selection: prediction of accuracy and maximisation of long term response. Genetica. 2008; 136: 245-257. Ref.: https://goo.gl/SpuwtD
- Habier D, Tetens J, Seefried FR, Lichtner P, Thaller G. The impact of genetic relationship information on genomic breeding values in German Holstein cattle. Genet Sel Evol. 2010; 42: 5. Ref.: https://goo.gl/QUYg71
- Hayes BJ, Macleod I, Daetwyler MD, Goddard ME. Towards genomic prediction from genome sequence data and the 1000 bull genomes project, Proceedings 4th International Conference on Quantiative Genetics, Edinburgh. 2012; O–54. Ref.: https://goo.gl/rg4DZJ
- Sanford JC, Klein TM, Wolf ED, Allen N. Delivery of substances into cells and tissues using a particle bombardment process. Journal of Particulate Science and Technology. 1987; 5: 27–37. Ref.: https://goo.gl/FXPGpK
- Klein RM, Wolf ED, Wu R, Sanford JC. High-velocity microprojectiles for delivering nucleic acids into living cells. Nature. 1987; 327: 70–73. Ref.: https://goo.gl/dApfMA
- Park F. Lentiviral vectors: are they the future of animal transgenesis? Physiol. Genomics. 2007; 31: 159–173. Ref.: https://goo.gl/2aqAeY
- Lee LY, Gelvin SB. T-DNA binary vectors and systems. Plant Physiol. 2008; 146: 325–332. Ref.: https://goo.gl/qamdgR
- Jackson DA, Symons RH, Berg P. Biochemical Method for Inserting New Genetic Information into DNA of Simian Virus 40: Circular SV40 DNA Molecules Containing Lambda Phage Genes and the Galactose Operon of Escherichia coli. PNAS. 1972; 69: 2904–2909. Ref.: https://goo.gl/YctKZY
- Brophy B, Smolenski G, Wheeler T, Wells D, L'Huillier P, et al. Cloned transgenic cattle produce milk with higher levels of β-casein and κ-casein. Nat Biotechnol. 2003; 21; 157–162. Ref.: https://goo.gl/J24QzX
- Clark J. The Mammary Gland as a Bioreactor: Expression, Processing, and Production of Recombinant Proteins. Journal of Mammary Gland Biology and Neoplasia. 1998; 3: 337–350. Ref.: https://goo.gl/EJfyn2
- Gordon K, Lee E, Vitale JA, Smith AE, Westphal H, et al. Production of human tissue plasmnogen activator in transgenic mouse milk. Biotechnology. 1987; 5: 1183-1187. Ref.: https://goo.gl/iqhpp6
- Anastasia B. Risk Assessment and Mitigation of AquAdvantage Salmon. 2010; ISB News Report. Ref.: https://goo.gl/Jjxcyw
- Thomas MA, Roemer GW, Donlan CJ, Dickson BG, Matocq M, et al. Ecology: Gene tweaking for conservation. Nature. 2013; 501: 485–486. Ref.: https://goo.gl/GtDny1
- Jaenisch R, Mintz B. Simian virus 40 DNA sequences in DNA of healthy adult mice derived from preimplantation blastocysts injected with viral DNA. Proc Natl Acad. 1974; 71: 1250–1254. Ref.: https://goo.gl/j3DBBS
- Sathasivam K, Hobbs C, Mangiarini L, Mahal A, Turmaine M, et al. Transgenic models of Huntington's disease. Philos Trans R Soc Lond B Biol Sci. 1999; 354: 963–969. Ref.: https://goo.gl/7LR7Jo
- Spencer LT, Humphries JE, Brantly ML; Transgenic Human Alpha 1-Antitrypsin Study Group. Antibody Response to Aerosolized Transgenic Human Alpha1-Antitrypsin. N Engl J Med. 2005; 352: 2030. Ref.: https://goo.gl/zgqVM4
- Schatten G, Mitalipov S. Developmental biology: Transgenic primate offspring. Nature. 2009; 459: 515–516. Ref.: https://goo.gl/nfzPnV
- Richard G. Genetically modified cows produce 'human' milk. 2011; Ref.: https://goo.gl/QaBjjC
- Wagner JS, McCracken J, Wells DN, Laible G, Targeted microRNA expression in dairy cattle directs production of -lactoglobulin-free, high-casein milk. Proceedings of the National Academy of Sciences. 2012; 109: 16811–16816. Ref.: https://goo.gl/oaZ6hT
- Margawati ET. Transgenic Animals: Their Benefits To Human Welfare. Actionbioscience. Retrieved June 29, 2014; Ref.: https://goo.gl/yvMECq
- Capecchi MR. Gene targeting in mice: functional analysis of the mammalian genome for the twenty-first century. Nat Rev Genet. 2005; 6: 507–512. Ref.: https://goo.gl/xeXiqP
- Cong L, Ran FA, Cox D, Lin S, Barretto R, et al. Multiplex genome engineering using CRISPR/Cas systems. Science. 2013; 339: 819–823. Ref.: https://goo.gl/QkraAU
- DiCarlo JE, Norville JE, Mali P, Rios X, Aach J, et al. Genome engineering in Saccharomyces cerevisiae using CRISPR-Cas systems. Nucleic Acids Res. 2013; 41: 4336–4343. Ref.: https://goo.gl/rT4FKq
- Mali P, Yang L, Esvelt KM, Aach J, Guell M, et al. RNA-guided human genome engineering via Cas9. Science. 2013; 339: 823–826. Ref.: https://goo.gl/keJNi3
- Friedland AE, Tzur YB, Esvelt KM, Colaiácovo MP, Church GM, et al. Heritable genome editing in C. elegans via a CRISPR-Cas9 system. Nat Methods. 2013; 10: 741–743. Ref.: https://goo.gl/QV1akB
- Xue H, Wu J, Li S, Rao MS, Liu Y. Genetic Modification in Human Pluripotent Stem Cells by Homologous Recombination and CRISPR/Cas9 System. Methods Mol Biol. 2016; 1307:173-190. Ref.: https://goo.gl/TtwWqh
- Esvelt KM, Wang HH. Genome-scale engineering for systems and synthetic biology. Mol Syst Biol. 2013; 9: 641. Ref.: https://goo.gl/yFaS15
- Ling MM, Robinson BH. Approaches to DNA mutagenesis: an overview, Analytical Biochemistry. 1997; 254: 157–178. Ref.: https://goo.gl/ayHjC4
- Capecchi MR. Altering the genome by homologous recombination. Science. 1989; 244: 1288-1292. Ref.: https://goo.gl/vZhv6s
- de Souza N. Primer: genome editing with engineered nucleases. Nat Meth. 2011; 9: 27-27. Ref.: https://goo.gl/zT5kkz
- Chevalier BS, Kortemme T, Chadsey MS, Baker D, Monnat RJ, et al. Design, Activity, and Structure of a Highly Specific Artificial Endonuclease. Molecular Cell. 2002; 10: 895-905. Ref.: https://goo.gl/GuDWgo
- Smith J, Grizot S, Arnould S, Duclert A, Epinat JC, et al. A combinatorial approach to create artificial homing endonucleases cleaving chosen sequences. Nucleic Acids Research. 2006; 34: e149. Ref.: https://goo.gl/KMHBAH
- Baker M. Gene-editing nucleases. Nat Meth. 2012; 9: 23-26. Ref.: https://goo.gl/qoViMw
- Urnov FD, Rebar EJ, Holmes MC, Zhang HS, Gregory PD. Genome editing with engineered zinc finger nucleases. Nat Rev Genet. 2010; 11: 636-646. Ref.: https://goo.gl/gYL6WE
- Boissel S, Jarjour J, Astrakhan A, Adey A, Gouble A, et al. megaTALs: a rare-cleaving nuclease architecture for therapeutic genome engineering. Nucleic Acids Research. 2014; 42: 2591–2601. Ref.: https://goo.gl/hEuSdm
- Bahrami A, Miraie-Ashtiani SR, Sadeghi M, Najafi A. miRNA-mRNA network involved in folliculogenesis interactome: systems biology approach. Reproduction. 2017; 154: 51-65. Ref.: https://goo.gl/cVfrhx
- Bahrami A, Miraie-Ashtiani SR, Sadeghi M, Najafi A, Ranjbar R. Dynamic modeling of folliculogenesis signaling pathways in the presence of miRNAs expression. J Ovarian Res. 2017; 10: 76. Ref.: https://goo.gl/LrNcDQ
- Alberghina L, Westerhoff HV. Systems Biology: Definitions and Perspectives. Topics in Current Genetics. 2005; 13: 13–30. Ref.: https://goo.gl/zYUL73
- Kholodenko BN, Sauro HM, eds. Systems Biology: Definitions and Perspectives. Topics in Current Genetics. 2005; 13: 357–451.
- Chiara R, Gerolamo L. Statistical Tools for Gene Expression Analysis and Systems Biology and Related Web Resources. In Stephen Krawetz, Bioinformatics for Systems Biology. 2009; Humana Press:. 181–205. Ref.: https://goo.gl/Jyuatn
- von Bertalanffy L. General System theory: Foundations, Development, Applications. George Braziller. 1976; 295. Ref.: https://goo.gl/d3kjVH
- Hodgkin AL, Huxley AF. A quantitative description of membrane current and its application to conduction and excitation in nerve. J Physiol. 1952; 117: 500–544. Ref.: https://goo.gl/KTq2ER
- Noble D. Cardiac action and pacemaker potentials based on the Hodgkin-Huxley equations. Nature.1960; 188: 495–497. Ref.: https://goo.gl/4w7HtK
- Rosen R. A Means toward a New Holism. Science. 1968; 161: 34–35. Ref.: https://goo.gl/USthvk
- Hunter P. Back down to Earth: Even if it has not yet lived up to its promises, systems biology has now matured and is about to deliver its first results. EMBO Reports. 2012; 13: 408–411. Ref.: https://goo.gl/7eoD7E
- Zeng BJ. On the concept of system biological engineering. Communication on Transgenic Animals. 1994a; 6.
- Zeng BJ. Transgenic animal expression system – transgenic egg plan (goldegg plan). Communication on Transgenic Animals. 1994b; 1:11
- Zeng BJ. From positive to synthetic science. Communication on Transgenic Animals. 1995; 11.
- Tomita M, Hashimoto K, Takahashi K, Shimizu TS, Matsuzaki Y, et al. E-CELL: Software Environment for Whole Cell Simulation,. Genome Inform Ser Workshop Genome Inform. 199; P 8: 147–155. Ref.: https://goo.gl/vRcbX5
- Karr JR, Sanghvi JC, Macklin DN, Gutschow MV, Jacobs JM, et al. A Whole-Cell Computational Model Predicts Phenotype from Genotype. Cell. 2012; 150: 389–401. Ref.: https://goo.gl/H9dwgF
- Tavassoly I. Dynamics of Cell Fate Decision Mediated by the Interplay of Autophagy and Apoptosis in Cancer Cells. Springer International Publishing. ISBN. 2015; 978-3-319-14961-5. Ref.: https://goo.gl/T5GmRj
- Nakano T. Molecular Communication. Cambridge. ISBN. 2013, 978-1-107-02308-6. Ref.: https://goo.gl/EsqF63
- Elowitz MB, Leibler S. A synthetic oscillatory network of transcriptional regulators. Nature. 2000; 403: 335–338. Ref.: https://goo.gl/Nw8FLz
- Gardner TS, Cantor CR, Collins JJ. Construction of a genetic toggle switch in Escherichia coli. Nature. 2000; 403: 339–342. Ref.: https://goo.gl/Bmkvyg
- Channon K, Bromley EH, Woolfson DN. Synthetic Biology through Biomolecular Design and Engineering. Curr Opin Struct Biol. 2008; 18: 491–498. Ref.: https://goo.gl/MpkfdD
- Stone M. Life Redesigned to Suit the Engineering Crowd. Microbe. 2006; 1: 566–570. Ref.: https://goo.gl/HkEBp2
- Zhang R, Lin Y. DEG 5.0, a database of essential genes in both prokaryotes and eukaryotes. Nucleic Acids Res. 2009; 37: D455–D458. Ref.: https://goo.gl/dmRreS
- Juhas M, Eberl L, Glass JI. Essence of life: Essential genes of minimal genomes. Trends Cell Biol. 2011; 21: 562–568. Ref.: https://goo.gl/zt5r8q
- Hutchison CA, Peterson SN, Gill SR, Cline RT, White O, et al., Global transposon mutagenesis and a minimal Myco- plasma genome. Science. 1999; 286: 2165–2169. Ref.: https://goo.gl/tU1oag
- Goodman AL, Wu M, Gordon JI. Identifying microbial fitness determinants by insertion sequencing using genome-wide transposon mutant libraries. Nat Protoc. 2011; 6: 1969 –1980. Ref.: https://goo.gl/oh2yj1
- van Opijnen T, Bodi KL, Camilli A. Tn-seq: High-throughput parallel sequencing for fitness and genetic interaction studies in microorganisms. Nat Methods. 2009; 6: 767–772. Ref.: https://goo.gl/p1bJZf
- Christen B, Abeliuk E, Collier JM, Kalogeraki VS, Passarelli B, et al. The essential genome of a bacterium. Mol Syst Biol. 2011; 7: 528. Ref.: https://goo.gl/PRdo5h
- Luo H, Lin Y, Gao F, Zhang CT, Zhang R. DEG 10, an update of the database of essential genes that includes both protein-coding genes and noncoding genomic elements. Nucleic Acids Res. 2014; 42: D574–D580. Ref.: https://goo.gl/15WnQ7
- Wetmore KM, Price MN, Waters RJ, Lamson JS, He J, et al. Rapid quantification of mutant fitness in diverse bacteria by sequencing randomly bar-coded transposons. MBio. 2015; 6: e00306-15. Ref.: https://goo.gl/cDDxcR
- Zhang R, Patena W, Armbruster U, Gang SS, Blum SR, et al. High-throughput genotyping of green algal mutants reveals random distribution of mutagenic insertion sites and endonucleolytic cleavage of transforming DNA. Plant Cell. 2014; 26: 1398–1409. Ref.: https://goo.gl/NwYcc2
- Angermayr SA, Gorchs Rovira A, Hellingwerf KJ. Metabolic engineering of cyanobacteria for the synthesis of commodity products. Trends Biotechnol. 2015; 33: 352–361. Ref.: https://goo.gl/QsVikZ
- Basulto D. Everything you need to know about why CRISPR is such a hot technology. Washington Post. 2015 Retrieved 5 December.
- Rollié S, Mangold M, Sundmacher K. Designing biological systems: Systems Engineering meets Synthetic Biology. Chemical Engineering Science. 2012; 69: 1–29. Ref.: https://goo.gl/AiVUFu
- Kaznessis YN. Models for synthetic biology. BMC Systems Biology. 2007; 1: 47. Ref.: https://goo.gl/ze2Sdr
- Masoudi-Nejad A, Bidkhori G2, Hosseini Ashtiani S2, Najafi A2, Bozorgmehr JH, et al. Cancer systems biology and modeling: microscopic scale and multiscale approaches. Semin. Cancer Biol. 2015; 30: 60–69. Ref.: https://goo.gl/LZwrco
- Najafi A, Bidkhori G, Bozorgmehr JH, Koch I, Masoudi-Nejad A. Genome scale modeling in systems biology: algorithms and resources. Curr. Genomics. 2014; 15: 130–159. Ref.: https://goo.gl/KXqKHi
- Kosuri S, Church GM. Large-scale de novo DNA synthesis: technologies and applications. Nature Methods. 2014; 11: 499–507. Ref.: https://goo.gl/SHRaZY
- Blight KJ, Kolykhalov AA, Rice CM. Efficient initiation of HCV RNA replication in cell culture. Science. 2000; 290: 1972–1974. Ref.: https://goo.gl/j344wQ
- Smith HO, Hutchison CA 3rd, Pfannkoch C, Venter JC. Generating a synthetic genome by whole genome assembly: {phi} X174 bacteriophage from synthetic oligonucleotides. Proc Natl Acad Sci USA. 2003; 100: 15440–15445. Ref.: https://goo.gl/ggr443
- Gibson DG, Benders GA, Andrews-Pfannkoch C, Denisova EA, Baden-Tillson H, et al. Complete chemical synthesis, assembly, and cloning of a Mycoplasma genitalium genome. Science.2008; 319: 1215–1220. Ref.: https://goo.gl/h3bj48
- Kramer BP, Fischer C, Fussenegger M. Biologic gates enable logical transcription control in mammalian cells. Biotechnol. Bioeng. 2004; 87: 478–484. Ref.: https://goo.gl/6NcbQL
- Nissim L, Bar-Ziv RH. A tunable dual-promoter integrator for targeting of cancer cells. Mol Syst Biol. 2010; 6: 444. Ref.: https://goo.gl/9KgZ27
- Lohmueller JJ, Armel TZ, Silver PA. A tunable zinc finger-based framework for Boolean logic computation in mammalian cells. Nucleic Acids Res. 2012; 40: 5180–5187. Ref.: https://goo.gl/aaS2eL
- Qi LS, Larson MH, Gilbert LA, Doudna JA, Weissman JS, et al. Repurposing CRISPR as an RNA-guided platform for sequence-specific control of gene expression. Cell. 2013, 152: 1173–1183. Ref.: https://goo.gl/xE5Pzc
- Maeder ML, Linder SJ, Cascio VM, Fu Y, Ho QH, et al. CRISPR RNA-guided activation of endogenous human genes. Nat Methods. 2013; 10: 977–979. Ref.: https://goo.gl/zHZdkq
- Kiani S, Beal J, Ebrahimkhani MR, Huh J, Hall RN, et al. CRISPR transcriptional repression devices and layered circuits in mammalian cells. Nat Methods. 2014; 11: 723–726. Ref.: https://goo.gl/EE86T4
- Nissim L, Perli SD, Fridkin A, Perez-Pinera P, Lu TK. Multiplexed and programmable regulation of gene networks with an integrated RNA and CRISPR/ CAS toolkit in human cells. Mol Cell. 2014; 54: 698–710. Ref.: https://goo.gl/LVgoyy
- Fussenegger M, Morris RP, Fux C, Rimann M, von Stockar B, et al. Streptogramin-based gene regulation systems for mammalian cells. Nat Biotechnol. 2000; 18: 1203–1208. Ref.: https://goo.gl/kGbh64
- Gillette MU, Sejnowski TJ. Physiology: biological clocks coordinately keep life on time. Science. 2005; 309: 1196–1198. Ref.: https://goo.gl/Bs48Gc
- Kaasik K, Lee CC. Reciprocal regulation of haem biosynthesis and the circadian clock in mammals. Nature. 2004; 430: 467–471. Ref.: https://goo.gl/YF6FNt
- Covert MW, Leung TH, Gaston JE, Baltimore D. Achieving stability of lipopolysaccharide-induced NF-kappa B activation. Science. 2005; 309: 1854–1857. Ref.: https://goo.gl/wCS2Qk
- Lahav G. The strength of indecisiveness: oscillatory behavior for better cell fate determination. Sci STKE. 2004; 55. Ref.: https://goo.gl/ufX2Y8
- Tigges M, Marquez-Lago TT, Stelling J, Fussenegger M. A tunable synthetic mammalian oscillator. Nature. 2009; 457: 309–312. Ref.: https://goo.gl/GseoBW
- Tigges M, Dénervaud N, Greber D, Stelling J, Fussenegger M. A synthetic low-frequency mammalian oscillator. Nucleic Acids Res. 2010; 38: 2702–2711. Ref.: https://goo.gl/SZy5fZ
- Stricker J, Cookson S, Bennett MR, Mather WH, Tsimring LS, et al. A fast, robust and tunable synthetic gene oscillator. Nature. 2008; 456: 516–519. Ref.: https://goo.gl/LPSYtK
- Ausländer S, Ausländer D, Müller M, Wieland M, Fussenegger M. Programmable single-cell mammalian biocomputers. Nature. 2012; 487: 123–127. Ref.: https://goo.gl/uU1EVR
- Montague MG, Lartigue C, Vashee S. Synthetic genomics: potential and limitations. Current Opinion in Biotechnology. 2012; 23: 659-665. Ref.: https://goo.gl/qa5AYx
- Deamer A. giant step towards artificial life? Trends Biotechnol. 2005; 23: 336–338. Ref.: https://goo.gl/4RkuQ6
- Malyshev DA, Dhami K, Lavergne T, Chen T, Dai N, et al. A semi-synthetic organism with an expanded genetic alphabet. Nature. 2014; 509: 385–388. Ref.: https://goo.gl/98PDPH
- Gibson DG, Glass JI, Lartigue C, Noskov VN, Chuang RY, et al. Creation of a Bacterial Cell Controlled by a Chemically Synthesized Genome. Science. 2010; 329: 52–56. Ref.: https://goo.gl/RTEw3p
- Rogers-Hayden T, Pidgeon N. Reflecting upon the UK’s Citizens’ Jury on Nanotechnologies: Nano Jury UK. Nanotechnology Law & Business. 2006; 167-178. Ref.: https://goo.gl/6dmCpM
- Wynne B. Creating Public Alienation: Expert Cultures of Risk and Ethics on GMOs. Sci Cult (Lond). 2001; 10: 445-481. Ref.: https://goo.gl/JWk4vh
- Gregory R, Fischhoff B, McDaniels T. Acceptable Input: Using Decision Analysis to Guide Public Policy Deliberations. Decision Analysis. 2005; 2: 4-16. Ref.: https://goo.gl/5hhYx2